Our team member, Shams Farhad, alongside her colleagues, has recently published a paper titled “Application of Hybrid Deep Learning Architectures for Identification of Individuals with Obsessive-Compulsive Disorder Based on EEG Data”
This study addresses the persistent challenge of accurately diagnosing Obsessive-Compulsive Disorder (OCD), a prevalent psychiatric condition characterized by intricate symptomatology often interwoven with other mental health disorders. Farhad and her colleagues’ research represents a significant leap forward, presenting a novel framework integrating advanced deep learning methodologies with electroencephalography (EEG) data to outline biomarkers for OCD diagnosis.
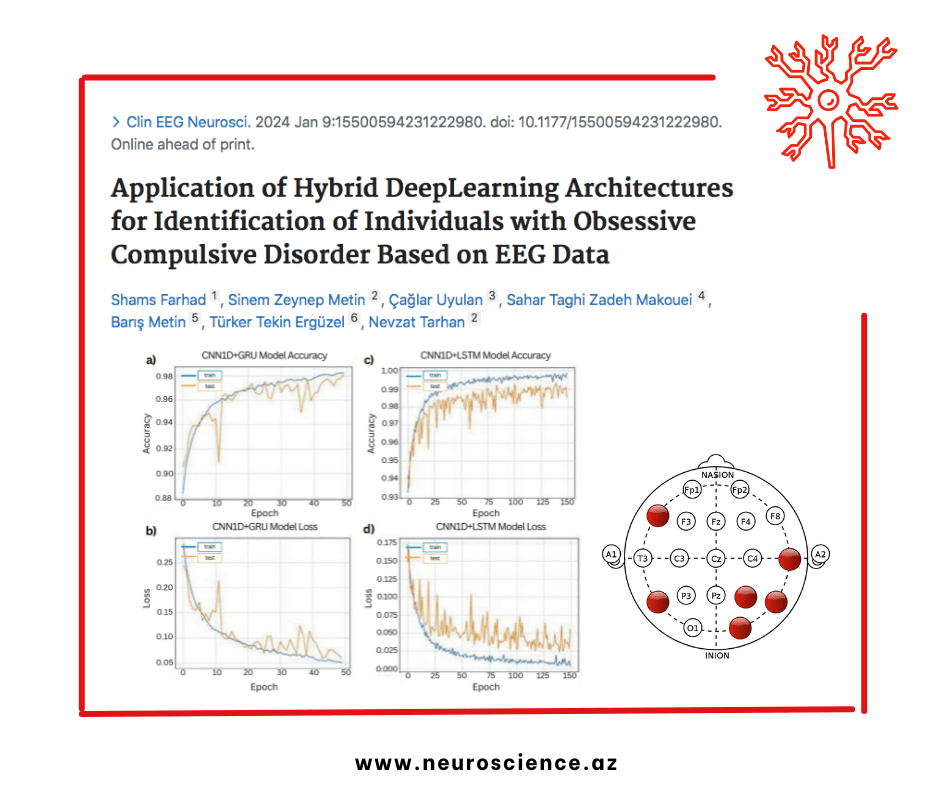
The study employed two hybrid deep learning models, combining one-dimensional convolutional neural networks (1DCNN) with either long-short term memory (LSTM) or gradient recurrent units (GRU). These models were trained to differentiate between individuals with OCD and healthy controls using EEG data.
Remarkably, both hybrid models demonstrated exceptional efficacy in distinguishing OCD patients from healthy counterparts. The 1DCNN-LSTM model exhibits a mean classification accuracy of 90.88%, while the 1DCNN-GRU model achieves 85.91% accuracy.
Moreover, the study identified specific brain regions—such as the inferior frontal, temporal, and occipital electrodes—as crucial in providing discriminative features for OCD diagnosis.
For more information: https://journals.sagepub.com/doi/10.1177/15500594231222980
Farhad S, Metin SZ, Uyulan Ç, Makouei STZ, Metin B, Ergüzel TT, Tarhan N. Application of Hybrid DeepLearning Architectures for Identification of Individuals with Obsessive Compulsive Disorder Based on EEG Data. Clin EEG Neurosci. 2024 Jan 9:15500594231222980. doi: 10.1177/15500594231222980. Epub ahead of print. PMID: 38192213.